Developing Data-driven Approach for Occupants-based Environmental Control
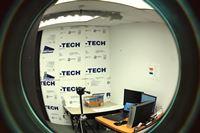
The design and operation of building systems frequently face conflicting goals between providing acceptable thermal comfort conditions and reducing building system’s relevant energy consumption. Integrating individually different occupants’ thermal comfort preferences into the building thermal environment control strategy has high potential to contribute to overcoming this conflict.
The goal of this study was to develop an intelligent control algorithm to maximize energy conservation efficiency while enhancing the occupants’ thermal comfort and satisfactions. Considering individual occupants’ different thermal preferences, two occupancy conditions were selected in this study: single-occupancy condition (SOC) and multi-occupancy condition (MOC). The SOC experiments were conducted to survey subjects’ thermal preference pattern while the thermal environmental conditions changed from 18ºC to 30ºC in the climate chamber. Meanwhile, subjects’ physical parameters were collected by heart rate sensors and survey forms to confirm the correlation between the indoor thermal condition and subjects’ individual features. With the consideration of real-time environment conditions and human individual features (such as gender, BMI, and heart rate), subjects’ individual thermal preference patterns were captured and learned by the machine learning algorithm.
The occupants’ thermal comfort preference under different environmental conditions can be predicted by the machine learning algorithm. Based on individuals’ thermal preference pattern, the Overall Thermal Dissatisfied (OTD) index was developed to determine the optimal set point temperature for minimizing the overall thermal dissatisfaction. The study result revealed the energy conservation potential up to 42% savings while significantly increasing occupants’ thermal comfort in a workplace environment.
keywords: Data-driven; Thermal comfort; Machine learning; Building system; Energy conservation
Add comment
Log in to post comments